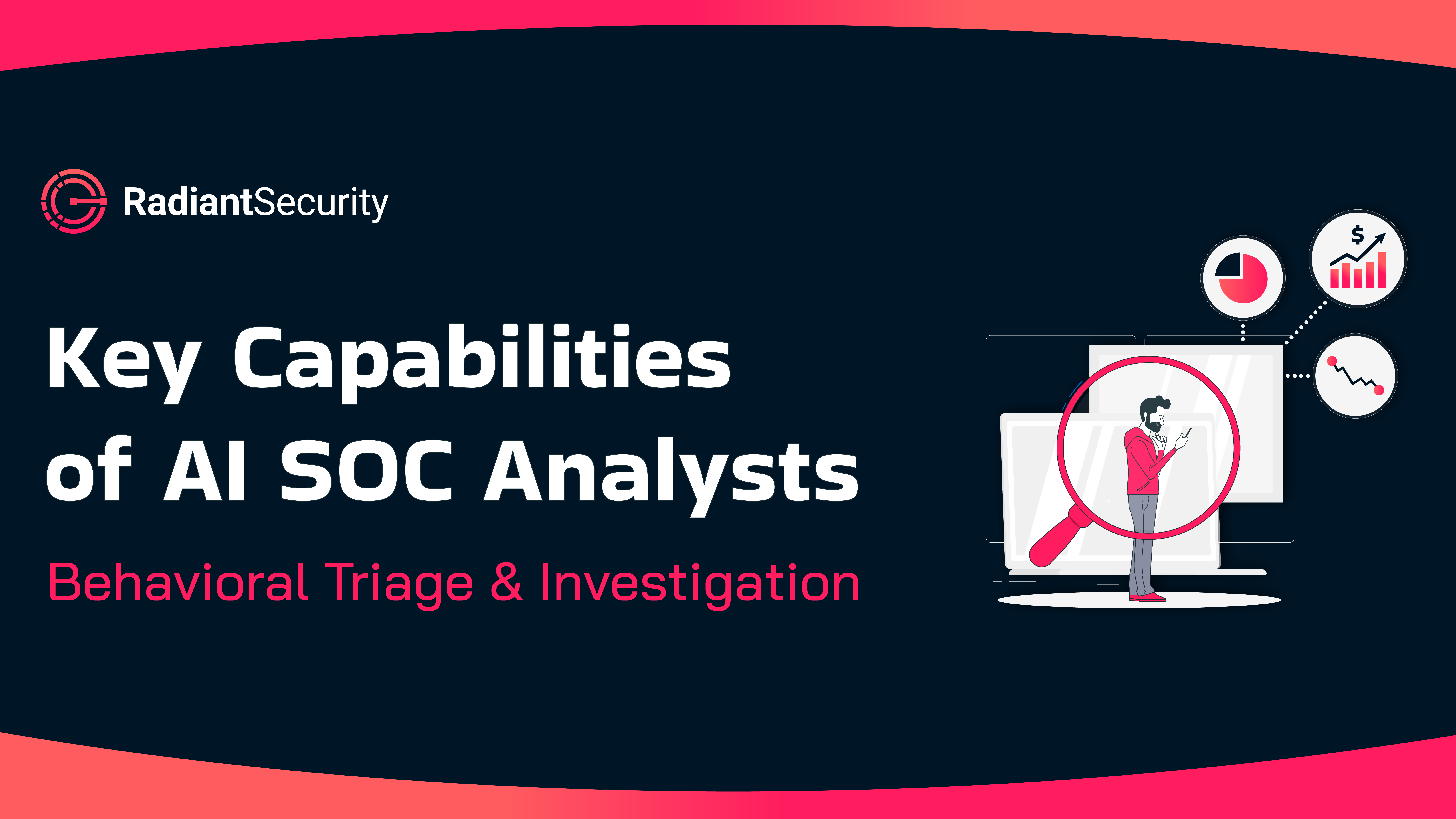
SOCs are under immense pressure, with 67% of cybersecurity leaders reporting their teams are understaffed (“ISC^2 Workforce Study 2023”). The demand for skilled security analysts continues to outpace supply, making traditional approaches to alert triage insufficient. Enter the AI SOC analyst: a solution designed to augment human staff, reduce false positives, and accelerate mean time to detect and respond to alerts. An AI SOC analyst can automate up to 90% of a Tier 1 analysts’ work.
This blog will focus on a core capability of the AI SOC analyst: behavioral investigation as well as how shifting the use of behavior analysis from detection to post detection can provide huge benefits to the SOC.
The Spotted History of Behavior Analysis in SecOps
Behavior analysis has long been a part of security operations, used in threat detection solutions like UEBA, XDR, and SIEM. The primary method of these solutions is to analyze and detect abnormal behavior patterns of user or machine activities in a corporate network, thus indicating a potential security incident such as a compromised credential or machine.
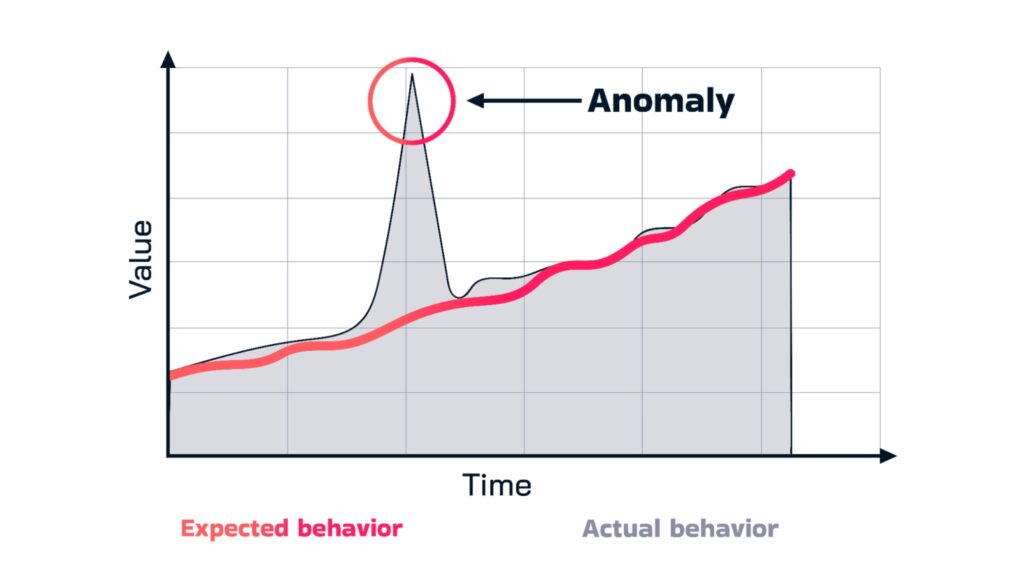
Figure 1 – An Illustration of a behavioral anomaly that may indicate a security incident.
While behavior analysis initially showed promise, it often created more challenges than solutions. Some significant challenges of this technology are:
- High Cost– standalone behavior analytics solutions were expensive, both in terms of annual fees and the compute resources required to run them.
- Tuning and Maintenance Headaches -These solutions also needed extensive tuning to optimize, which often demanded dedicated staff to operationalize.
- Negative Impact to Productivity – of easing the workload of SOC analysts, behavior analysis frequently added to it by generating large volumes of alerts, including many false positives. SOC teams, already overwhelmed by staff shortages, found themselves with even more work, and often, up to 90% of alerts went ignored by overburdened analysts, allowing legitimate attacks to slip through the cracks.
Despite these drawbacks, behavior analysis has become a common technique embraced by virtually every category of security solution which performs threat detection.
What is Behavioral Triage & Investigation
Behavioral triage and investigation reimagines behavior analysis specifically for post-detection workflows, where understanding how the activity in an alert compares with behavioral baselines can provide immediate, high-fidelity insights into the maliciousness of an alert, as well as its scope and root cause. Instead of attempting to detect anomalies in real-time like previous generations of behavior analysis, which often creates false positives, behavioral investigation analyzes user and device activity after a potential threat has been flagged by a security alert.
To solve the problem of low-fidelity results, behavioral analysis must be used more selectively, and later in the SecOps process. Used as a primary detection signal, behavior analysis often finds too many anomalies because employees do abnormal things with astounding regularity. However, asking the same question as part of triage or investigation provides the benefit of scoping the analysis down to a smaller data set, such as “only the users who entered their credentials into a phishing site”, and thus producing higher fidelity results.
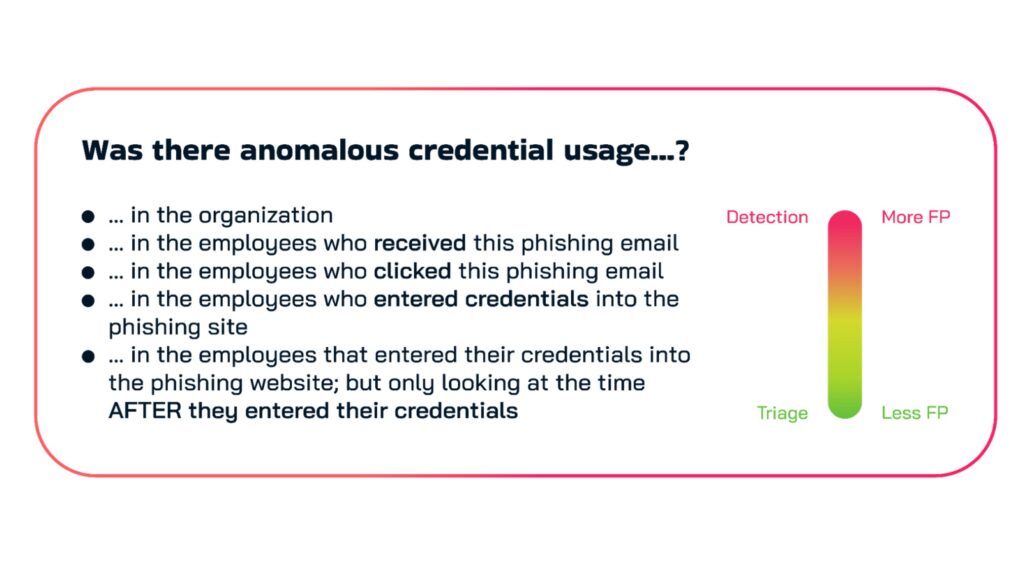
Figure 2 – An example showing how behavior analytics can have different levels of fidelity depending on where in the process it is used.
Moreover, behavioral inspection is a powerful technique that can be used during the triage and investigation process, however until recently its use in post-detection activities was reserved for only the most critical alerts or forensic analysis tasks. This is because SOCs often lack ready access to the required information (behavioral baselines) and the time or skill required to create them on demand (by querying and interpreting existing security data)—at scale.
Behavior Analysis | Behavioral Investigation | |
Stage | Pre-detection | Post-Detection |
Impact on SOC productivity | Negative – it creates alerts which must be investigated, and increases work backlogs. | Positive – it helps analysts resolve existing security alerts and reduces work backlogs. |
Fidelity | Low | High |
Figure 3 – A summary comparison of behavioral analysis vs behavioral investigation.
Why AI Analysts are perfect for this task
AI SOC analysts are constantly learning — security best practices, investigation techniques, knowledge bases, response tactics, attack trends, and even activity baselines for monitored environments. This data ends up in a rich data fabric, which the AI engine can utilize during triage and investigation, to obtain much needed context and to answer investigative questions. This essentially provides AI analysts with deep familiarity with the monitored environment that rivals or surpasses that of human employees working in the SOC.
Let’s look at a few examples of where this type of data can be useful.
Example 1 – Triaging Impossible Travel Alerts
A common alert that often has false positives is that of impossible travel. These identity alerts attempt to identify compromised credentials in use by detecting when credentials are used concurrently in multiple locations or in a timeframe that could not be possible given the constraints of human travel technology (e.g. a login in New York and then five minutes later in Singapore.)
An AI analyst can use it’s behavioral baselines during triage of an impossible travel alert to answer contextual questions like:
- Is it normal for this user (or their peers) to travel to this location?
- Is this normal login behavior?
- Are they using their normal device?
- Are they using a proxy or VPN? Is that normal for the user?
- Etc.
Each of these questions provides clues about the possible maliciousness of the alert.
Example 2 – Triaging DLP Alerts
DLP alerts are notoriously difficult and time-consuming to triage because they require context and information that SOC analysts typically don’t have. Analysts often need to contact business stakeholders to determine if the actions in a specific DLP alert—like Kate downloading reports from Salesforce or Oliver uploading data to an application—are acceptable.
An AI analyst can streamline this process by using behavioral data to understand context, eliminating the need to ask these questions. By comparing the activity in question to the behavioral baseline of the organization, peers, or the user, the AI analyst can triage and investigate alerts more efficiently and more accurately. This reduces the need for back-and-forth communication, shortening the time to reach a conclusion and enabling faster response actions.
Seeing A Richer Picture of Attacks
Shifting behavior analytics from pre-detection to post-detection offers a significant benefit: it enables a more comprehensive story during impact and root cause analysis. For instance, consider a phishing attack that steals credentials. By identifying anomalous activity after the credentials are compromised, you can track the apps used and actions taken during malicious sessions. This not only provides a complete picture of the attack but also allows for more effective containment and response measures, resulting in a more thorough resolution.
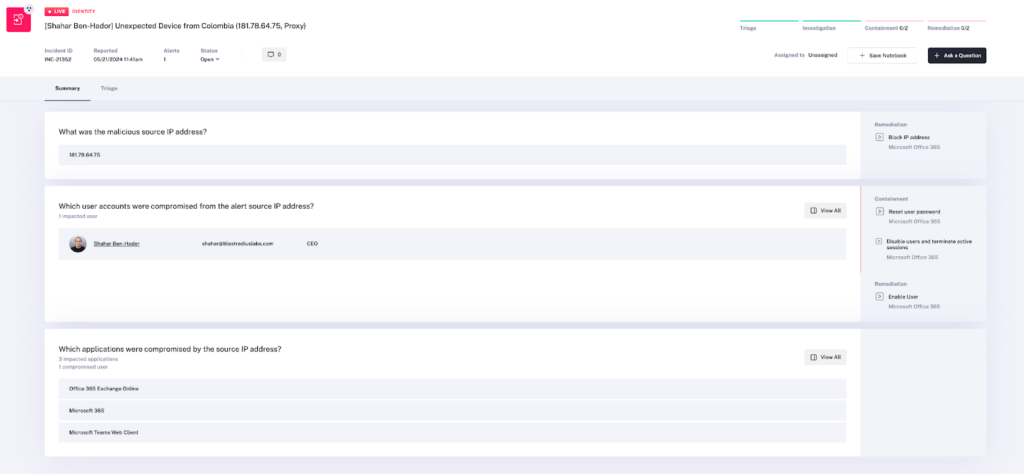
Figure 4 – An AI generated response plan showing the compromised applications that were impacted as part of credential compromise.
Want to see our AI-Powered SOC Analysts in action? View our product tour at radiantsecurity.ai, no form fill required.